
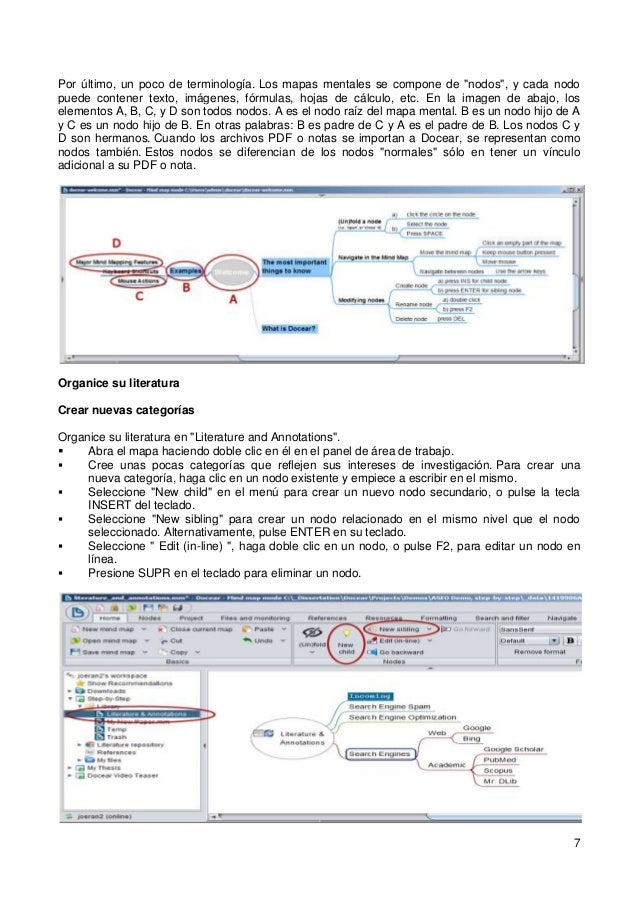
The main aim of our paper is to predict the popularity of a person using ML classifiers. Furthermore, our proposed strategy includes personalized suggestions and customer expertise, in addition to addressing multi-disciplinary concerns. Using a publicly available dataset, we found that our proposed method outperformed previous uncertain methods in terms of overall performance and the capacity to return relevant, valuable, and quality publications at the top of the recommendation list. Thus, a novel collaborative approach is proposed that provides better performance.
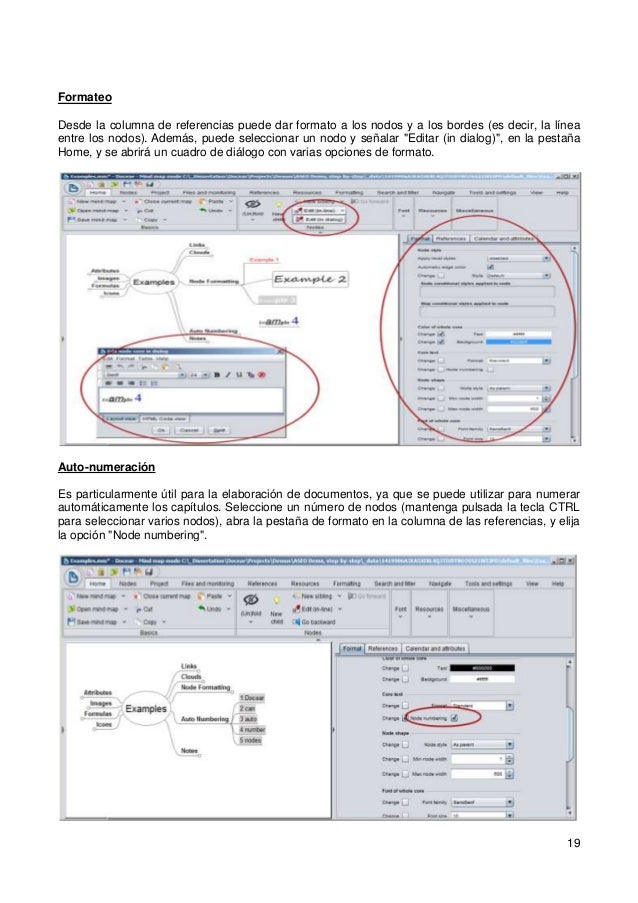
The proposed system, RPRSCA, is unique and gives personalized recommendations irrespective of the research subject. We make use of contextual metadata that are publicly available to gather hidden relationships between research papers in order to personalize recommendations by exploiting the advantages of collaborative filtering. This work demonstrates an efficient model, known as RPRSCA: Research Paper Recommendation System Using Effective Collaborative Approach, to address these uncertain systems for the recommendation of quality research papers. However, due to several issues, such as copyright constraints, these methodologies assume that the recommended articles’ contents are entirely openly accessible, which is not necessarily the case. Recommenders for research papers have appeared over the last decade to make it easier to find publications associated with the field of researchers’ interests. It aims at helping users find things online by offering recommendations that closely fit their interests. The Artificial Intelligence Recommender System has emerged as a significant research interest. Our method achieves the highest standardized accuracy score of 87.26% (with Cliff’s δ = 0.88 at 99.999% confidence level) and 42.7% with the automatically transformed linear baseline model.
#Docear review 2016 software
Given the software description of a newly envisioned software, our tool yields an effort estimate for developing it. Our software description similarity model is basically a machine learning model trained using the PVA on the software product descriptions of GitHub repositories. The significant contributions of our article are (i) novel SDEE software metrics derived from developer activity information of various software repositories, (ii) an SDEE dataset comprising the SDEE metrics’ values derived from approximately 13,000 GitHub repositories from 150 different software categories, and (iii) an effort estimation tool based on SDEE metrics and a software description similarity model. We propose an efficient SDEE method for open source software, which provides accurate and fast effort estimates. As such, the existing SDEE methods suffer from low usage and accuracy. Most organizations do not have access to sufficient and reliable forms of such data from past projects. Software development effort estimation (SDEE) generally involves leveraging the information about the effort spent in developing similar software in the past. Briefly, the contributions of this paper are as follows: (1) providing an overview of the prevailing scenario in a range of application domains associated with IoT based recommender systems, (2) providing a framework of some real-world applications, (3) outlining key areas for the improvement towards context-aware personalized services in the future research, and (4) providing a bibliometric trend analysis prevalent in the concerned domain. Therefore, the aim of this paper is to study the present practices, approaches, and challenges in the aforementioned domain along with possible solutions. In recent years, the focus of researchers has drifted towards utilizing recommender systems to improve offered selections to IoT users. However, researchers are now exploring the potential of IoT systems in other domains, one of them being recommender systems. Smart applications of this scenario provide assistance and services to the users in smart homes, smart cities, smart retail, agriculture, healthcare, etc. This domain is gaining popularity worldwide because of the wave it has brought in both research and industrial sector.
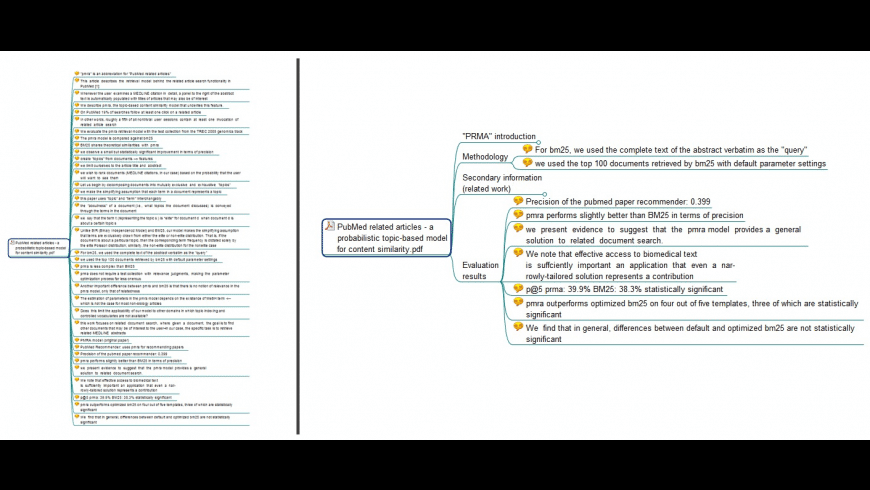
Typically, Internet of Things (IoT) is perceived as the technology for connecting people, devices, vehicles, home appliances, etc., and 212 billion heterogenous devices are presumed to be connected by 2020 under its umbrella.
